How to Implement AI Predictive Analytics in Healthcare: Top Benefits & Use Cases
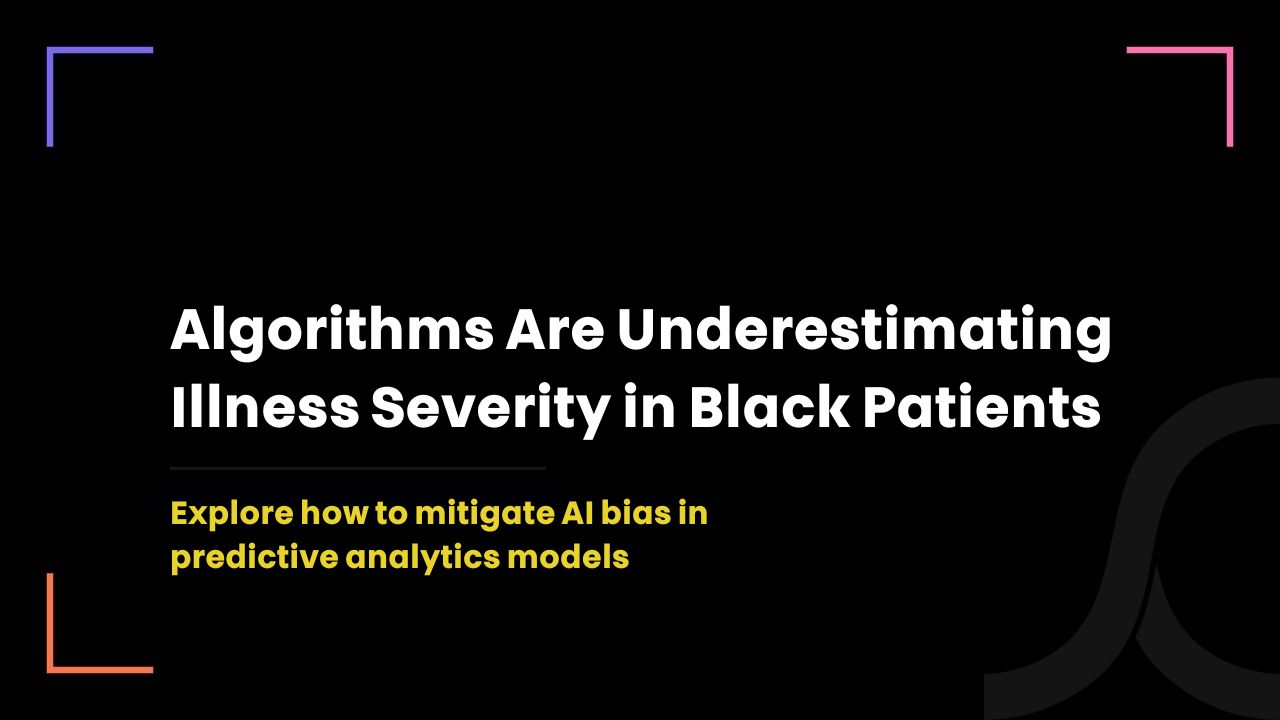
2 months ago
Canadian hospitals are under pressure to improve patient care, cut costs, and streamline operations.
In today’s fast-paced environment, reacting to problems as they arise is no longer enough.
Hospitals need to predict and prepare for future challenges to deliver the best care.
This is where AI predictive analytic models come into play.
These models use data to forecast trends, spot at-risk patients, and optimize resources. By shifting from reactive to proactive care, hospitals can stay ahead in meeting modern healthcare demands.
For example, 65% of hospitals now use AI or predictive models.
The most common applications include predicting patient health outcomes (92%) and identifying high-risk patients (79%).
Unlike older methods that grouped patients by broad factors like income, predictive analytics uses healthcare data and algorithms to create highly accurate, personalized insights.
With challenges like an aging population, rising chronic diseases, and strained facilities, traditional methods often fall short. Canada’s health services rank 10th out of 11 high-income countries, with gaps in access, efficiency, and outcomes.
Predictive analytics offers hospitals a chance to close these gaps and transform healthcare delivery.
Understanding Predictive Analytics
Predictive analytics is transforming how hospitals operate. It helps them anticipate future needs and take proactive steps to improve care, save costs, and run more efficiently. Let’s break down what it’s all about.
What Are Predictive Models?
Predictive models are smart tools that analyze data using statistical formulas and algorithms. They find patterns and trends to forecast future events, helping hospitals make better decisions.
For example, these models can:
- Predict patient readmissions.
- Identify individuals at risk for chronic diseases.
- Forecast the demand for hospital beds.
They use data from electronic health records (EHRs), medical imaging, and patient demographics to provide accurate insights. This enables hospitals to plan ahead, reduce costs, and enhance patient care.
How Do They Work?
Predictive models use large datasets and advanced machine learning (ML) algorithms to identify patterns.
- Neural networks, a type of ML, can detect complex links between factors like medical history, demographics, and visit patterns.
- These models analyze trends in admissions, length of stay, and discharges to forecast demand.
They constantly update as new data comes in, turning complex information into clear, actionable insights. This allows hospitals to make timely decisions and provide better patient management.
How Are They Different from Older Models?
Older models grouped patients based on non-medical data like zip codes or buying habits. These methods worked for general trends but lacked precision.
Predictive models are different because:
- They use healthcare-specific data, like patient history and medical codes.
- They focus on individual needs instead of grouping people into broad categories.
- They analyze millions of data points to deliver more accurate predictions.
This shift makes predictive analytics far more effective in addressing healthcare challenges.
What Are the Benefits?
Predictive analytics offers hospitals a range of advantages:
- Strategic Planning: Helps hospitals plan for future needs, improve care, and manage costs.
- Improved Patient Care: Identifies at-risk patients for early interventions and better outcomes.
- Cost Savings: Reduces unnecessary treatments, prevents readmissions, and optimizes resources.
- Efficiency: Helps manage beds, staffing, and discharges more effectively.
- Proactive Approach: Anticipates demand, minimizes overcrowding, and improves workflows.
- Data-Driven Decisions: Empowers hospitals with actionable insights to improve care delivery.
By adopting predictive analytics, hospitals can move from reacting to problems to preventing them, ensuring better care and smoother operations.

Key Areas Where Predictive Analytics Transforms Healthcare
Predictive analytics is like a crystal ball for healthcare, offering powerful insights that help hospitals run better and deliver exceptional care. Let’s break it down into areas where it makes a big impact:
1. Understanding Patient Health Trends: Predictive models can forecast how a patient's health might change during their hospital stay. This helps doctors plan treatments and resources more efficiently.
2. Spotting High-Risk Patients: AI tools can flag outpatients who might need extra care. Early intervention prevents issues like hospital readmissions and keeps patients healthier.
3. Better Appointment Scheduling By analyzing past data, predictive analytics reduces wait times and no-show appointments. This makes clinics more efficient and keeps patients happy.
4. Smarter Bed Management: Hospitals can predict admissions and discharges. This ensures beds are available when needed, avoiding long waits.
5. Planning for No-Shows: AI can predict who might miss their appointment. Hospitals can adjust schedules to prevent wasted time and resources.
6. Allocating Resources: From medical staff to equipment, predictive analytics ensures resources are available where they’re needed most.
7. Managing Supplies: Hospitals can avoid shortages or wastage by forecasting supply needs, like medications and equipment.
8. Guiding Clinical Decisions: AI offers quick insights for doctors to choose the best treatments, saving time and improving outcomes.
9. Enhancing Population Health: Predictive analytics identifies trends in diseases or health risks within a community. Hospitals can act early to improve overall health.
10. Supporting Value-Based Care: By improving care quality and reducing costs, predictive analytics helps hospitals achieve value-based care goals.
11. Reducing Readmissions: AI highlights patients likely to return to the hospital, enabling better post-discharge plans and fewer readmissions.
12. Personalized Treatments: Each patient is unique. Predictive analytics helps create tailored treatment plans based on genetics, lifestyle, and health history.
13. Avoiding Surgical Complications: Predictive tools flag patients at risk for complications during surgery. This means better pre- and post-op care.
By using predictive analytics, hospitals can anticipate challenges, reduce costs, and make healthcare more personal and proactive. It’s the future of smarter, patient-centered care.
Get a personalized, Free Technology Assessment to explore the right solutions for your hospital’s unique needs.
A Simple Guide to Implementing Predictive Analytics in Healthcare
Implementing predictive analytics in healthcare requires a thoughtful and step-by-step approach. Here’s a simple breakdown to help you get started:
1. Define Clear Goals
- Focus on specific problems predictive analytics can solve, like improving patient scheduling or predicting readmissions.
- Start with easy-to-implement use cases that fit into current workflows.
- Set measurable goals to track progress.
2. Collect and Prepare Data
- Gather data from electronic health records (EHRs), imaging, or patient histories.
- Ensure the data is clean, accurate, and complete.
- Protect patient privacy with robust security measures and compliance with regulations like PIPEDA/HIPAA.
3. Choose the Right Models
- Select AI or machine learning (ML) models that fit your goals, such as forecasting patient risks or optimizing bed management.
- Keep models understandable for better trust and transparency.
4. Build and Test Models
- Develop models tailored to your specific needs using diverse data.
- Test their performance to ensure accuracy and reliability.
- Check for any bias in the data to maintain fairness.
5. Integrate Models into Workflows
- Make the models a part of daily operations without disrupting healthcare teams.
- Present insights in a simple, actionable way that helps providers make decisions.
6. Train and Launch
- Train staff to understand and use the predictive tools effectively.
- Deploy the models in real clinical settings and gather feedback to refine them.

Overcoming Challenges in Predictive Analytics Implementation
Implementing predictive analytics in healthcare is exciting, but it does come with challenges. Here’s how to tackle the most common ones:
1. Breaking Down Data Silos
Challenge: Healthcare data is often stuck in separate systems across departments, making it hard to create a full picture of patient health.
Impact: Predictive models miss out on important information, leading to less reliable predictions.
Solution:
- Use tools to connect different data systems so they can "talk" to each other.
- Set up clear rules for sharing and accessing data securely.
- Encourage collaboration between departments to build a culture of sharing.
2. Building Trust in AI Algorithms
Challenge: Doctors and patients can be wary of AI because they don’t fully understand how it works.
Impact: Mistrust can block AI adoption and limit its potential to improve care.
Solution:
- Involve clinicians in the design and testing of AI models to align them with real-world practices.
- Use Explainable AI (XAI) to show how predictions are made.
- Gather feedback from patients and clinicians to ensure their needs are addressed.
3. Fighting Bias in AI
Only 44% of U.S. hospitals using AI evaluate their models for bias, risking inequitable care.
Challenge: AI models can reflect biases in their training data, leading to unfair outcomes for certain groups.
Impact: Biases in AI can cause misdiagnoses and health disparities, like a U.S. algorithm that underestimated illness severity in Black patients compared to white patients.
Solution:
- Use diverse, representative data to train AI models.
- Regularly audit AI systems for bias and correct them when necessary.
- Include social, economic, and demographic data to account for health inequities.
By addressing these challenges, healthcare organizations can maximize the benefits of predictive analytics and ensure equitable, high-quality patient care.
