Healthcare Data Lakes Implementation and Maintenance ( Including Top 6 Benefits)
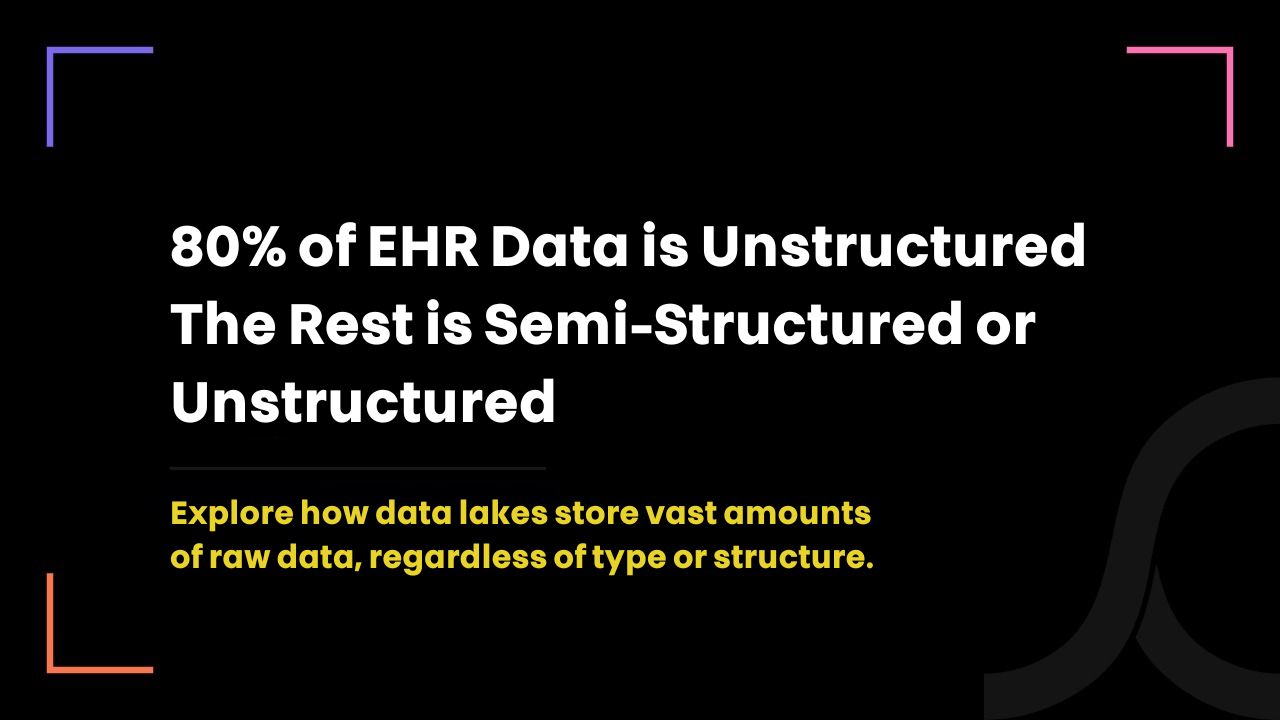
2 months ago
Healthcare data is expanding at a staggering pace.
From electronic health records (EHRs) to wearable devices and genomic research, the volume of healthcare data surpassed 35 zettabytes in 2020 and is projected to reach 2,314 exabytes by 2025.
For example, a single patient's records can generate over 50 terabytes of data, and a mid-sized hospital with 500 beds manages about 50 petabytes every year.
While this influx of data holds great potential, it also presents challenges.
Healthcare data often exists in silos, trapped in separate systems.
Traditional databases struggle with the variety of structured and unstructured data – like medical images or clinician notes.
Only 20% of EHR data is structured, with the rest in semi-structured or unstructured formats.
This is where data lakes come in.
Data lakes store vast amounts of data in its raw form, regardless of type or structure.
They break down data silos and allow healthcare organizations to store, integrate, and analyze data in one place.
With data lakes, healthcare organizations can unlock powerful insights, improving patient care and driving innovation.
Defining Data Lakes: What They Are & How They Differ
A data lake is a centralized repository that can store vast amounts of data in its raw, original format. Unlike traditional storage systems, a data lake doesn’t require data to be processed or organized before it's stored.
This makes it perfect for capturing and preserving all kinds of data – whether structured, semi-structured, or unstructured.
In healthcare, data lakes are especially useful because only about 20% of Electronic Health Record (EHR) data is structured. The rest, including things like doctors’ notes, medical images, and lab reports, is semi-structured or unstructured.
A data lake can handle all this diverse information. It can store data from sources like IoT sensors, patient records, social media feeds, and even videos.
So, how do data lakes differ from traditional systems like data warehouses?
1. Data Storage: Data lakes store raw, unprocessed data in its original format, while data warehouses store pre-processed, structured data that has been transformed to fit a specific schema.
2. Schema Application: Data lakes use a "schema-on-read" approach. This means the structure of the data isn’t defined until it’s needed. This offers more flexibility for analysis. In contrast, data warehouses use a "schema-on-write" approach, where data must fit a predefined structure before it can be stored.
3. Flexibility and Scalability: Data lakes are highly flexible and scalable. They can handle huge amounts of data, even petabytes, and adapt to different analytical needs without extensive transformations. Data warehouses are more rigid, optimized for consistent reporting but not as agile when dealing with large, diverse datasets.
In healthcare, where data comes in many forms, data lakes offer a better way to manage and analyze all this information efficiently.

The Benefits of Data Lakes for Healthcare Organizations
1. Improved Data Integration
Data lakes consolidate information from various sources—like electronic health records (EHRs), patient-reported data, and even smart devices—into one unified platform.
This integration fosters seamless collaboration between hospitals, insurance companies, and public health authorities.
For instance, Phoenix Children’s Hospital integrated data from 40 systems into their data lake, significantly enhancing patient care and operational efficiency.
2. Enhanced Analytics and Decision-Making
With raw data stored in its native format, data lakes support advanced analytics, real-time analysis, and predictive modeling.
These capabilities eliminate the constraints of traditional systems and improve data accessibility by up to 89%, leading to faster, more informed decision-making.
3. Better Patient Care
By providing a comprehensive view of patient data, data lakes help create detailed profiles, identify high-risk patients, and enable personalized treatment plans. They also support real-time monitoring, contributing to improved health outcomes and proactive healthcare delivery.
4. Cost-Efficiency
Cloud-based data lakes reduce reliance on expensive on-premise infrastructure, cutting costs associated with hardware, maintenance, and data transfers by up to 71%.
They also provide secure and compliant storage for electronic protected health information (ePHI), making them a cost-effective alternative to traditional storage solutions.
5. Operational Improvements
Data lakes enhance operational efficiency by optimizing provider performance, resource allocation, and logistics management. They also analyze public data, like social media trends, to aid in service planning and better resource distribution.
6. Accelerated Research and Innovation
Vast and diverse datasets drive advancements in medical research and innovation. Data lakes help researchers identify trends, generate hypotheses, and develop new treatments faster. This accelerates breakthroughs in healthcare, supporting the development of cutting-edge therapies and medications.

Real-World Applications of Data Lakes in Healthcare
Data lakes are transforming healthcare by addressing challenges and enabling better outcomes. Here are some impactful applications:
1. Phoenix Children’s Hospital
By integrating data from 40 systems, the hospital created tools like a kidney care dashboard and medication dosing algorithms, improving patient care and operational efficiency.
2. Predicting Healthcare Costs
Data lakes merge EHRs, patient records, and external data to build accurate cost models, such as predicting cardiovascular risks.
3. Evidence-Based Care
Integrating clinical research, genomics, and EMRs enables data-driven, personalized care models.
4. Improving Patient Outcomes
Data lakes create a holistic view of patient data, supporting personalized treatments and predictive care models for conditions like diabetes.
5. Real-Time Monitoring
Continuous data analysis allows real-time vital tracking, reducing response times in critical care.
6. Customized Health Plans
Insurers analyze claims, social data, and IoT device inputs to design tailored health plans.
7. Early Disease Detection
Machine learning on data lakes helps identify diseases early, like detecting heart conditions from heart sound recordings.
8. Addressing SDOH
Socioeconomic and clinical data integration enables targeted interventions to address health disparities.
9. Better Medical Decisions
Access to comprehensive data uncovers trends and improves decision-making at the point of care.

Implementing and Maintaining a Data Lake in Healthcare
Building a data lake is just the start—it needs proper care and management to remain useful. Without attention, it can turn into a “data swamp,” making it hard to find or use valuable information. Let’s dive into the key steps to implement and maintain a data lake in healthcare.
1. Keep It Organized
- Metadata Tagging: Label all incoming data with tags. This makes it easy to find and analyze later.
- Terminology Management: Use consistent terms across the organization to avoid confusion.
- Data Quality Checks: Regularly review the data to ensure it’s accurate, complete, and useful.
2. Ensure Strong Governance and Security
- Data Governance: Set clear policies for managing and using the data.
- Privacy and Security: Use advanced tools like blockchain and secure authentication to protect sensitive patient information.
- Compliance: Follow regulations like PIPEDA or HIPAA to ensure data is stored and shared responsibly.
Related: Top 10 PIPEDA Canada Myths Busted
3. Build the Right Foundation
- Cloud vs. On-Premises: Decide whether to use a cloud platform for scalability or an on-premises server for more control.
- Big Data Architecture: Adopt modern data architectures to handle large datasets effectively.
4. Rely on Experts
Managing a data lake requires skilled professionals:
- Data Scientists: To extract meaningful insights from raw data.
- Analysts: To clean and interpret the data for healthcare decisions.
- IT Teams: To maintain and secure the system.
5. Introducing the Data Lakehouse
A data lakehouse is a modern approach that combines the flexibility of a data lake with the structure of a data warehouse. Here’s why it’s great for healthcare:
- It supports real-time analytics and dashboards for quick decision-making.
- It integrates seamlessly with cloud services, making it scalable and cost-efficient.
- It’s perfect for AI and machine learning, enabling predictive healthcare solutions.
By maintaining a clean, secure, and well-organized data lake, healthcare organizations can unlock new opportunities to improve patient care, make smarter decisions, and innovate faster.
How we turn your healthcare data lake into a smooth-running machine
With over 10 years of experience in healthcare IT, we’re here to help Canadian healthcare organizations build data lakes that actually work — and yes, we make sure they’re PIPEDA-compliant too (because who wants a compliance headache, right?).
Our team of experts knows healthcare data inside and out — from securing it to making sense of it. We make sure all your data sources play nice together, giving you analytics and AI insights that actually do something useful.
Need to ensure your data is secure, governed, and ready to make smarter decisions? We’ve got you covered from start to finish.
Let’s talk and see how we (IT Service Provide in Canada) can turn your data lakes into smooth-running machines that improve both efficiency and patient outcomes.