Healthcare Data Warehouse Architecture & Implementation: A Strategic Playbook (2025)
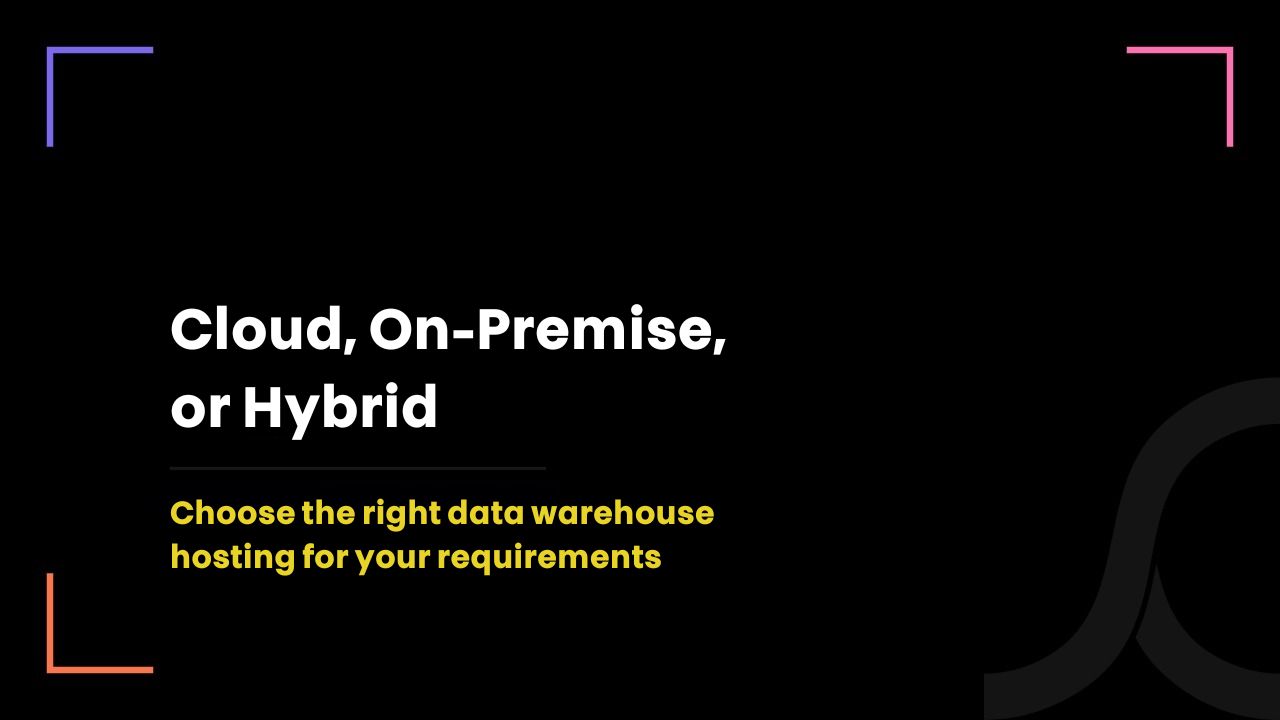
2 months ago
The healthcare industry is generating more data than ever.
By 2025, data from electronic health records, insurance claims, and other sources is expected to grow by 36%.
But here’s the catch—most healthcare organizations struggle to manage and use this data effectively.
Did you know nearly 80% of clinics don’t fully use digital tools?
That means they miss out on valuable insights from patient data.
So, how can healthcare providers turn this data into better care and smarter decisions?
Well, the answer lies in healthcare data warehousing.
In this blog, we’ll walk you through a simple, step-by-step guide to building a healthcare data warehouse.
We’ll cover everything—from setting clear goals to ensuring data security and compliance.
Let’s explore how you can manage your data better in 2024 and beyond!
What is a Healthcare Data Warehouse?
A healthcare data warehouse (DWH) is like a central storage hub for all your organization’s data. It collects and organizes information from various sources like:
- Electronic health records (EHRs)
- Lab results
- Insurance claims
- Financial data
- Research data
Unlike systems that only focus on clinical data, a DWH gives you the bigger picture.
It standardizes and structures the data, making it easy to analyze and report on. This helps healthcare providers uncover insights and make smarter decisions.
How Data Management Has Changed Over Time
Healthcare data management has come a long way.
Remember the days of paper files? Those are gone.
Now, we’ve moved from simple EHR systems to big data analytics and cloud storage. Here’s what changed:
- Before: Data was scattered across different systems. It was hard to find and even harder to analyze.
- Now: Centralized data warehouses bring all that information together in one place.
Big data and advanced technology made this shift possible.
And with the push for value-based care, the need for better data management is greater than ever.
The Current State of Healthcare Data
The healthcare industry is drowning in data. In fact, it accounts for about one-third of all the world’s data. And this number is only growing.
By 2025, data from electronic health records (EHRs), insurance claims, and billing systems is expected to grow by 36%.
That’s a massive increase!
The healthcare big data market, which was worth $11.5 billion in 2016, is projected to hit $70 billion by 2025.
But here’s the problem: despite having so much data, many clinics aren’t using it effectively.
About 80% of clinics still don’t fully leverage digital tools.
This means they’re missing out on valuable insights that could improve patient care and operations.
That’s why implementing a healthcare data warehouse is so important.
It helps organizations make sense of all this data and turn it into actionable insights.
How Healthcare Data Warehousing Transforms Care
A healthcare data warehouse isn’t just about storing data—it’s a game-changer for modern healthcare. Here’s how it makes a difference:
1. Better Patient Care
A data warehouse gives you a complete view of a patient’s history.
This helps doctors make more informed decisions and create personalized treatment plans. The result? Better outcomes for patients.
2. Smarter Decision-Making
By analyzing trends and patterns, healthcare organizations can predict future needs.
This makes planning and resource allocation more efficient.
3. More Efficient Operations
Tasks like billing, claims, and reporting become much easier.
This reduces admin work and cuts costs, freeing up time to focus on patients.
4. Support for Value-Based Care
Data warehouses help providers compare costs and outcomes.
This makes it easier to improve care quality while managing expenses.
5. Boost for Medical Research
Researchers can use anonymized data to study trends, run clinical trials, and even develop new treatments.
Types of Healthcare Data Warehouses
Not all data warehouses are the same. Here are the main types:
Individual Data Marts
- Focus on specific areas like finance or clinical operations.
- Faster to set up but may cause issues when integrating data across departments.
Enterprise Data Warehouses
- Combine all data into one system for a complete, integrated view.
- More complex to set up but offers better scalability and insights.
Organizations can also mix both approaches or choose between on-premise, cloud-based, or hybrid systems, depending on their needs.
Architecting a Healthcare Data Warehouse: Major Components
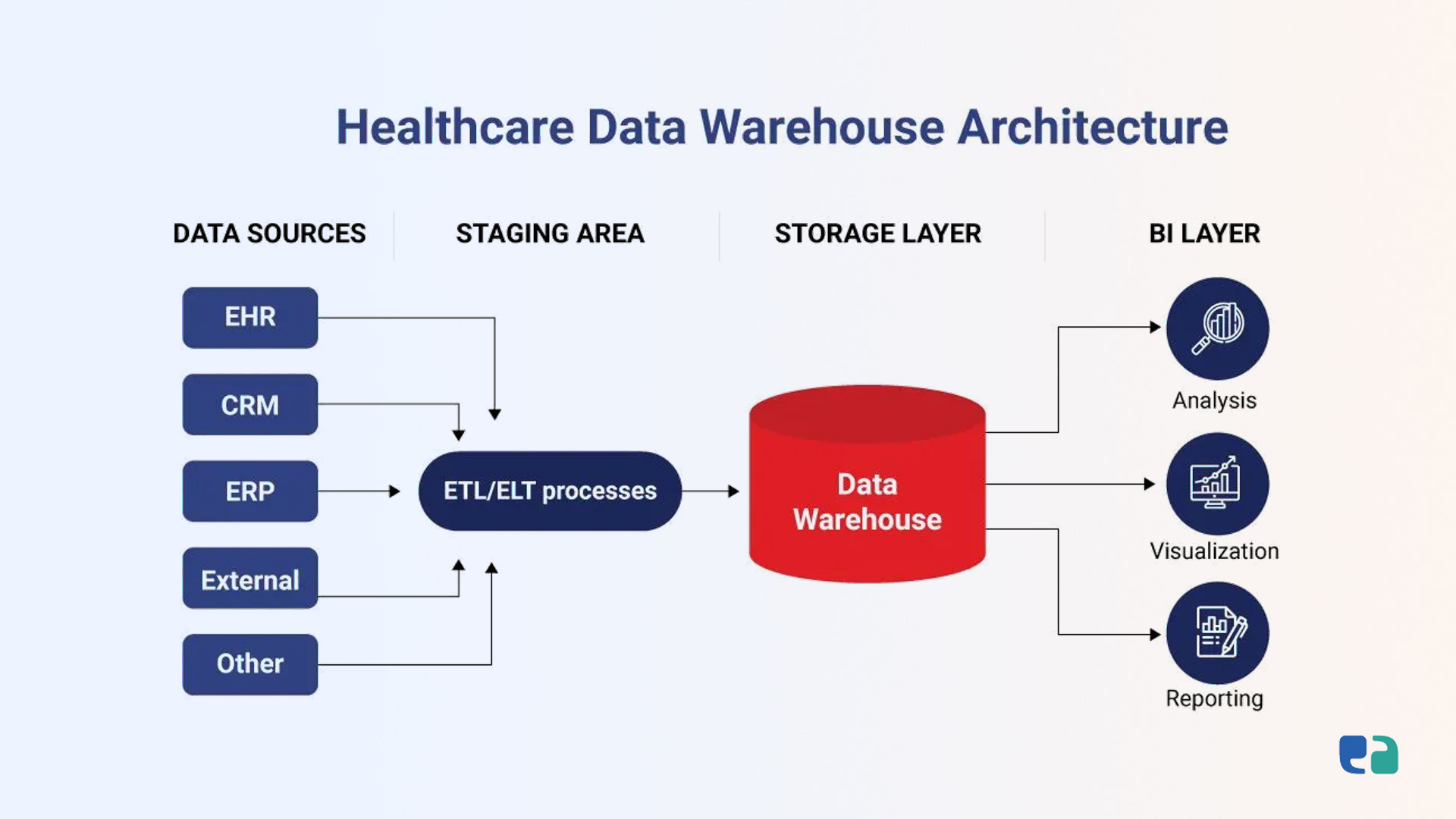
Building a healthcare data warehouse is like assembling a puzzle. Each layer plays an important role in managing and analyzing data. Here’s a breakdown of the key components:
1. Data Source Layer
This is where the journey begins. Data comes from various systems like:
- EHR/EMR systems: Patient records, medical history, and treatment details.
- Lab systems: Blood tests and diagnostic results.
- Pharmacy systems: Medication dispensing and prescription details.
- Medical imaging systems: X-rays, MRIs, and other scans.
- Insurance systems: Claims and billing data.
- IoT devices: Wearables tracking heart rate, sleep, and activity levels.
- Patient portals: Appointment schedules and communication preferences.
These sources feed raw, unprocessed data into the warehouse.
2. Staging Area
The staging area is like a data "clean-up" zone. Here’s what happens:
- Data validation: Errors are fixed, and missing information is addressed.
- Quality checks: Data is reviewed to ensure it’s accurate and consistent.
- Temporary storage: Data is stored briefly as it’s prepared for the next stage.
This step ensures only reliable data enters the warehouse.
3. Storage Layer
This is the core of the warehouse, where data is stored long-term. Key features include:
- Data modeling: Data is organized in ways (like star or snowflake schemas) to make analysis easier.
- Partitioning: Large datasets are broken into smaller pieces, improving speed and efficiency.
- Data marts: Specific sections of data are created for departments like finance or clinical operations.
The storage layer ensures data is accessible and easy to analyze.
4. Analytics and Presentation Layer
This is where the magic happens—turning raw data into actionable insights:
- Reports: Customizable tools create detailed reports tailored to user needs.
- Dashboards: Interactive charts and graphs highlight trends and patterns.
- Advanced analytics: Integration with machine learning tools predicts trends and supports proactive care.
This layer empowers users to make data-driven decisions effortlessly.
Choosing the Right Healthcare Data Warehouse Model
Implementing a healthcare data warehouse (DWH) isn't one-size-fits-all.
It depends on your organization’s goals, resources, and growth plans. Here’s a quick guide to the main approaches and options:
1. Individual Data Mart Approach
This model focuses on smaller, department-specific data hubs, called data marts. For example, you might first create one for finance analytics and later add one for patient care.
- Why choose it? It’s quick, cost-effective, and ideal for smaller projects.
- Challenges? Data silos may form, and integrating these marts can get tricky.
2. Enterprise-Wide Data Warehouse
This is the go-big approach. It creates a central repository for all your data, offering a unified view across your organization.
- Why choose it? It provides integrated data for better decision-making and supports large-scale analytics.
- Challenges? It takes time, resources, and careful planning to set up.
3. Hybrid Model
Can’t pick one? The hybrid model combines both. Start small with data marts and slowly merge them into a bigger warehouse.
- Why choose it? It’s flexible and can grow with your organization.
- Challenges? It needs strategic planning to avoid integration headaches.
Deployment Options
Decide where to host your data warehouse:
- Cloud: Flexible and scalable with pay-as-you-go pricing. Great for organizations without large IT budgets.
- On-Premise: Keeps everything on your own servers. Perfect for maximum control but involves higher setup costs.
- Hybrid: A mix of both. Store sensitive data on-site and use the cloud for other tasks like backups.
Scaling for Growth
As data grows, your warehouse must keep up:
- Vertical scaling: Add more power (CPU, memory) to existing servers.
- Horizontal scaling: Add more servers to distribute the workload.
- Cloud scaling: Adjust resources on-demand with flexible cloud platforms.
Smooth Migration
Migrating from old systems? Here’s the process:
1. Assess data: Review what needs to be moved and its quality.
2. Clean and transform: Fix and format data to fit the new system.
3. Load data: Transfer it to the new warehouse.
4. Validate: Check everything for accuracy.
5. Cutover: Switch to the new system with minimal disruption.
Choosing the right model and strategy can make all the difference in building a successful data warehouse. Start small or go big—what matters is finding what fits your needs best.

Data Integration and Standardization: The First Step in Building a Strong Healthcare Data Warehouse
Creating a medical data warehouse (DWH) requires two key steps: integrating and standardizing data. These ensure data from various sources is combined and prepared for easy analysis and reporting.
ETL vs. ELT: Getting the Data Ready
There are two main methods to get data into the DWH: ETL (Extract, Transform, Load) and ELT (Extract, Load, Transform).
- ETL involves pulling data from different sources, cleaning and transforming it, and then loading it into the warehouse. It ensures that data is standardized and ready for analysis.
- ELT is a bit faster. It loads data first and transforms it later inside the DWH. This method takes advantage of powerful cloud platforms, making it more flexible and efficient for large data volumes.
Choosing between ETL and ELT depends on how complex your data is and how much data you’re working with.
Healthcare Data Standards: Ensuring Consistency
Healthcare data comes in many formats, so standardizing it is crucial. Some common standards include:
- HL7/FHIR: These standards allow different healthcare systems to share information easily.
- SNOMED CT: A global system used to represent medical terms.
- ICD-10: Used to classify diseases and injuries.
- LOINC: Helps standardize lab and clinical test results.
Using these standards helps different systems communicate and share data without confusion, making data more reliable and useful.

Making Data Work: Mapping, Transformation, and Real-Time Integration
Once data is integrated, the next step is to make sure it's organized and accurate, ready for meaningful analysis.
Data Mapping and Transformation: Making Sense of the Data
Data mapping connects data from various sources to the DWH. This involves:
- Data cleansing: Fixing errors or inconsistencies in the data.
- Deduplication: Removing duplicate records.
- Format conversion: Changing data formats to match DWH standards.
By ensuring data is mapped and transformed correctly, we make sure that everything in the DWH is accurate and useful.
Master Data Management: A Single Source of Truth
Master data management (MDM) keeps everything consistent by maintaining a central place for key data, like patient or provider information.
This reduces redundancy and ensures data accuracy across all systems.
Real-Time Integration: Keeping Data Fresh
In healthcare, up-to-date data is crucial. Real-time integration lets the DWH receive data as it comes in. For example:
- Patient monitoring: Data from wearable devices can be sent in real time, allowing doctors to monitor patients remotely.
- Alerts: If there’s an issue like abnormal test results, the DWH can send an alert right away, so doctors can act fast.
- Dashboards: Real-time data helps administrators make quick decisions, like allocating resources or managing patient flow.
With real-time integration, healthcare organizations can make faster, more informed decisions and provide better care.
Healthcare Data Warehouse Examples
Here are some real-world examples of how healthcare organizations leverage data warehouses to optimize operations, enhance decision-making, and improve patient care:
1. OhioHealth Data Warehouse
OhioHealth implemented an enterprise data warehouse by purchasing a pre-built system.
This centralized repository enables business users to easily access, analyze, and act on critical data, streamlining decision-making processes and improving overall operational efficiency.
2. Global Health Observatory (GHO) by the World Health Organization
The GHO is a global health observatory that provides comprehensive health data, tools, and analysis.
Its robust data warehouse supports public health professionals worldwide by offering insights into a wide range of topics, from mortality rates to healthcare systems, empowering them to track and improve global health metrics.
3. National Clinical Data Repository (NCDR) in Ukraine
As part of Ukraine's national eHealth initiative, the NCDR plays a critical role in addressing the inefficiencies of traditional paper-based systems.
The healthcare data warehouse incorporates advanced data security features, including pseudonymization, access control, and interoperability via FHIR standards, ensuring the integrity and privacy of health data.
Security and Compliance in Healthcare Data Warehousing
In healthcare data warehousing, protecting patient data is a must. PHIPA & HIPAA set strict rules to ensure PHI stays secure. For example, data storage, access control, and transmission must be tightly controlled.
Data Encryption Methods
Encryption protects data by making it unreadable without a key.
- In transit: For instance, using TLS/SSL to secure data when it's sent over the internet.
- At rest: When data is stored, it's encrypted with algorithms like AES to protect it from unauthorized access.
Access Control
Access to PHI is strictly regulated in Canada.
- Role-based access control (RBAC) restricts data access based on roles. For instance, a nurse can view patient records relevant to their care, but administrative staff cannot.
- Multi-factor authentication (MFA) adds extra security, requiring users to confirm their identity with both a password and a one-time code.
Audit Trails
Tracking data access is essential. Audit logs record who accessed data, when, and what actions they took. This helps spot security issues and ensures PHIPA/HIPAA compliance.
Backup and Privacy
Backups protect data against loss, and privacy-preserving techniques like anonymization help safeguard identities.
Healthcare organizations must also stay compliant with international regulations like the GDPR when operating globally.

Benefits and Use Cases of Data Warehousing in Healthcare
Clinical Benefits
Data warehouses improve patient care by integrating data from various sources, helping providers identify issues early for proactive, personalized care, like Phoenix Children’s Malnutrition App.
They also support clinical decision-making by offering timely access to patient data, improving diagnosis and treatment accuracy, as seen with St. Luke’s University Health Network’s use of data for value-based care.
Operational Benefits
They optimize resource use, reduce costs, and monitor key performance indicators like wait times and readmission rates.
Data warehouses also help with capacity planning by predicting future healthcare needs.
Research Benefits
Data warehouses streamline clinical trials, medical research, and drug development by providing centralized data for analysis and identifying disease patterns.
Financial Benefits
They improve revenue cycle management, streamline claims processing, and help detect fraud.
Data warehouses also contribute to cost containment by identifying inefficiencies and optimizing resource use.
Advanced Analytics in Healthcare Data Warehousing
Advanced analytics can greatly enhance healthcare data warehousing, offering valuable insights for better care.
1. Machine Learning: Machine learning predicts health outcomes, like Phoenix Children’s Malnutrition App, which identifies at-risk patients. It also helps prioritize care by stratifying patient risk levels.
2. Natural Language Processing (NLP): NLP analyzes unstructured data, such as doctor’s notes, turning it into actionable insights and uncovering hidden trends.
3. Computer Vision: Computer vision analyzes medical images like X-rays and MRIs, aiding faster diagnoses and detecting abnormalities.
4. Time Series Analysis: Tracking data over time helps understand disease progression and treatment effectiveness, optimizing care and resources.
5. Pattern Recognition: Pattern recognition detects trends, identifies risk factors, and even helps detect healthcare fraud.
By integrating these techniques, healthcare data warehouses can improve patient care, reduce costs, and boost operational efficiency.
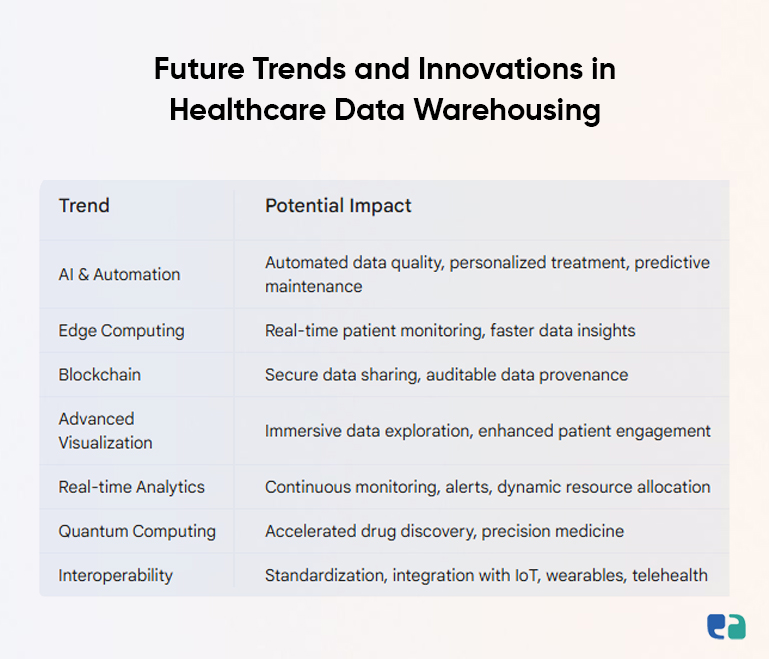
Challenges and Solutions in Healthcare Data Warehousing
1. Technical Challenges
- Data Volume: Healthcare data is growing rapidly.
- Solution: Use cloud-based data warehouses for scalability and cost efficiency.
- Performance Issues: Quick data access is essential.
- Solution: Build scalable systems and use cloud services to ensure fast queries.
- System Integration: Integrating different data sources can be tricky.
- Solution: Use tools like FHIR and ETL processes to standardize data.
- Legacy Systems: Older systems may not fit with new setups.
- Solution: Build custom APIs and interfaces for smooth integration.

2. Organizational Challenges
- Change Management: Resistance to data-driven culture.
Solution: Engage stakeholders early and involve business leaders.
- Staff Training: Employees need training to use the system.
Solution: Offer comprehensive training programs.
- Resource Allocation: Sufficient budget and resources are needed.
Solution: Plan carefully and use cloud solutions to optimize resources.
- Stakeholder Alignment: Ensure all stakeholders understand the project’s goals.
Solution: Communicate value and involve key decision-makers.
3. Data Quality Challenges
- Standardization: Data from different sources may vary.
Solution: Implement standards like FHIR for consistency.
- Missing Data: Incomplete data can affect accuracy.
Solution: Regular audits and data governance practices.
- Duplicate Data: Duplicate records can cause issues.
Solution: Use tools like Master Patient Index (MPI) to eliminate duplicates.
- Inconsistencies: Inconsistent data can affect integrity.
Solution: Establish data governance policies to ensure consistency.
